How Netflix’s Recommendation Engine Drives Success
Netflix's recommendation engine is a powerful tool that keeps users engaged by offering personalised content. Learn how this helped Netflix with retention.
In the world of streaming, Netflix reigns supreme, captivating millions of users worldwide. One of the most critical components of its success is its Recommendation Engine—a powerful growth hacking tool that has not only kept viewers hooked but also significantly improved user retention. This article explores how Netflix leverages algorithms and user data to deliver personalized content and how other platforms can adopt similar strategies to boost user growth.
The Power of Personalization: Netflix’s Secret Weapon
Netflix operates in a saturated market, with hundreds of competitors vying for attention. However, what sets Netflix apart is its highly personalized approach to content recommendations. The platform relies on a sophisticated algorithm that suggests movies, series, and documentaries tailored to individual preferences, creating a unique experience for each user.
The personalization is so effective that over 80% of the content watched on Netflix comes from recommendations. But how does Netflix achieve this level of accuracy?
How Netflix’s Recommendation Engine Works
Netflix’s recommendation engine leverages machine learning algorithms, data analytics, and user behavior tracking to provide highly relevant suggestions. Below are the key components that power the engine:
User Data Collection Netflix collects vast amounts of data from its uses
- Watch history (e.g., what content a user has watched, rewatched, or skipped).
- Search queries (e.g., what titles a user searches for).
- Viewing habits (e.g., when and how long users watch, whether they binge or consume content slowly).
- Ratings and interactions (e.g., thumbs up/down, adding content to a watchlist).
This data provides insight into user preferences and trends.
Collaborative Filtering Netflix compares users with similar tastes using collaborative filtering. For example, if two users share a high percentage of watched titles, the system will recommend shows that one user has seen but the other has not. This method creates a community-based recommendation system, improving content discovery.
Content-Based Filtering In this method, Netflix analyzes the attributes of a show or movie, such as genre, cast, director, and length. It then suggests content with similar attributes based on a user’s previous viewing choices. This approach helps create a personalized content library that feels curated just for you.
Contextual Information Netflix doesn't only rely on what users watch but also when they watch it. For example, a user may prefer comedies on weekends and documentaries on weekdays. The recommendation engine adapts its suggestions based on the time of day, day of the week, and even the device being used (e.g., mobile vs. smart TV).
A/B Testing Netflix continuously runs A/B tests to see what works best for users. For example, they test different thumbnails for the same content and track which image leads to more clicks. By fine-tuning its user experience with data, Netflix increases engagement and reduces churn.
How Netflix’s Recommendation Engine Drives Growth
Netflix’s recommendation system is not just a cool feature—it’s a growth hack that drives user retention and engagement. Here's how:
- Reducing Decision Fatigue: Netflix’s algorithm minimizes the overwhelming experience of choosing from thousands of titles by delivering a refined selection. This keeps users on the platform longer.
- Encouraging Binge-Watching: By offering tailored suggestions, Netflix ensures that after watching one episode or movie, the user is immediately presented with content they are likely to enjoy. This leads to more viewing sessions and, ultimately, higher retention.
- Keeping Users in the Ecosystem: Netflix continually adapts its content suggestions based on real-time data. Users are less likely to seek out other streaming platforms when they feel that Netflix "knows" their tastes better than any competitor.
Lessons for Other Platforms: How to Use Data to Grow Your User Base
Netflix’s approach to personalization can be applied across various industries to drive growth. Here’s how other platforms can leverage data to engage users and foster retention:
1. Utilize Machine Learning for Personalization
- E-commerce platforms can use machine learning to recommend products based on purchase history, browsing behavior, and preferences. Personalized product suggestions not only boost sales but also improve customer satisfaction.
- Music streaming services like Spotify already use similar algorithms to curate personalized playlists. Any platform offering a large variety of content can leverage personalization to guide users toward content they’ll enjoy.
2. Analyze User Behavior in Real Time
Understanding user behavior in real time allows platforms to adapt quickly. For example, a fitness app might suggest a specific workout routine based on the user’s activity levels during a particular time of day, just like Netflix suggests content based on time of use.
Content websites can use behavioral data to serve personalized articles or blog posts based on the reader's past interests, keeping them engaged longer on the platform.
3. Reduce Cognitive Load with Smart Recommendations
Users are overwhelmed by choice, whether it’s entertainment, shopping, or content. By using collaborative filtering and data-driven recommendations, business can reduce the friction involved in decision-making and keep users engaged. Amazon, for example, thrives on personalized product recommendations and ‘Customers Also Bought’ features.
4. Improve Engagement with Dynamic Content
Just as Netflix experiments with thumbnails and content descriptions to maximize clicks, platforms can A/B test dynamic elements like:
- Product images and descriptions in e-commerce
- Article thumbnails in content platforms.
- User interface elements in mobile apps to see which versions increase user engagement.
5. Leverage Contextual Data for Deeper Personalization
Platforms can incorporate contextual information like device type, location, or time of day to optimize recommendations. For example, a food delivery app might suggest lighter meals for lunchtime and heartier meals for dinner based on user habits.
6. Adopt a Data-First Culture
Netflix's growth is fueled by its commitment to data-driven decision-making. Platforms across industries must adopt a data-first culture, using metrics to drive product features, UX changes, and customer engagement strategies. Regularly collecting and analyzing data will enable companies to adapt quickly to user preferences.
Conclusion
Netflix’s recommendation engine is a textbook example of growth hacking at scale. By leveraging algorithms, user data, and continuous testing, Netflix has created an environment where users feel seen and understood, driving engagement and retention.
Any platform—whether in e-commerce, content, or services—can benefit from Netflix’s approach to data. The key is to focus on delivering personalized experiences that make users feel valued, reducing decision fatigue, and continually refining the user experience based on data. By doing so, platforms can not only grow their user base but also create loyal, long-term customers.
Ready to unlock exponential growth for your business? CONTACT US
Latest post
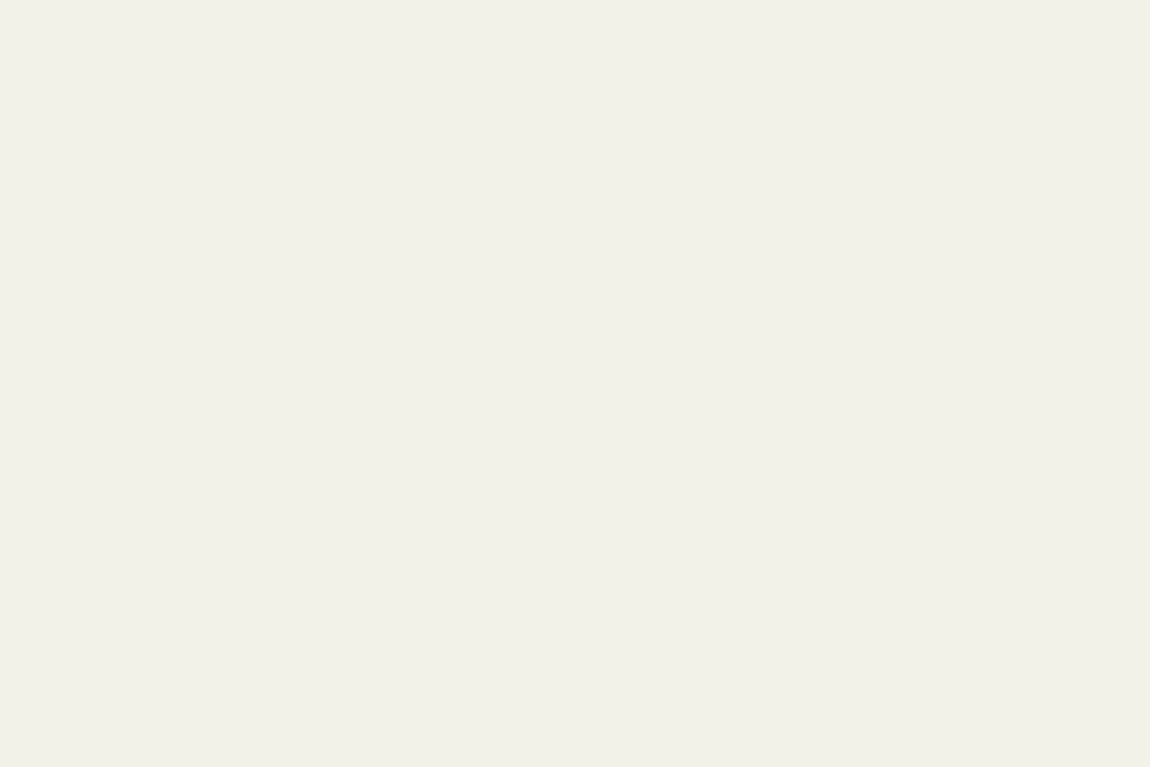
Looking to start a business in Australia? Discover 20 top small business ideas, from online startups to service-based ventures, and turn your idea into reality!
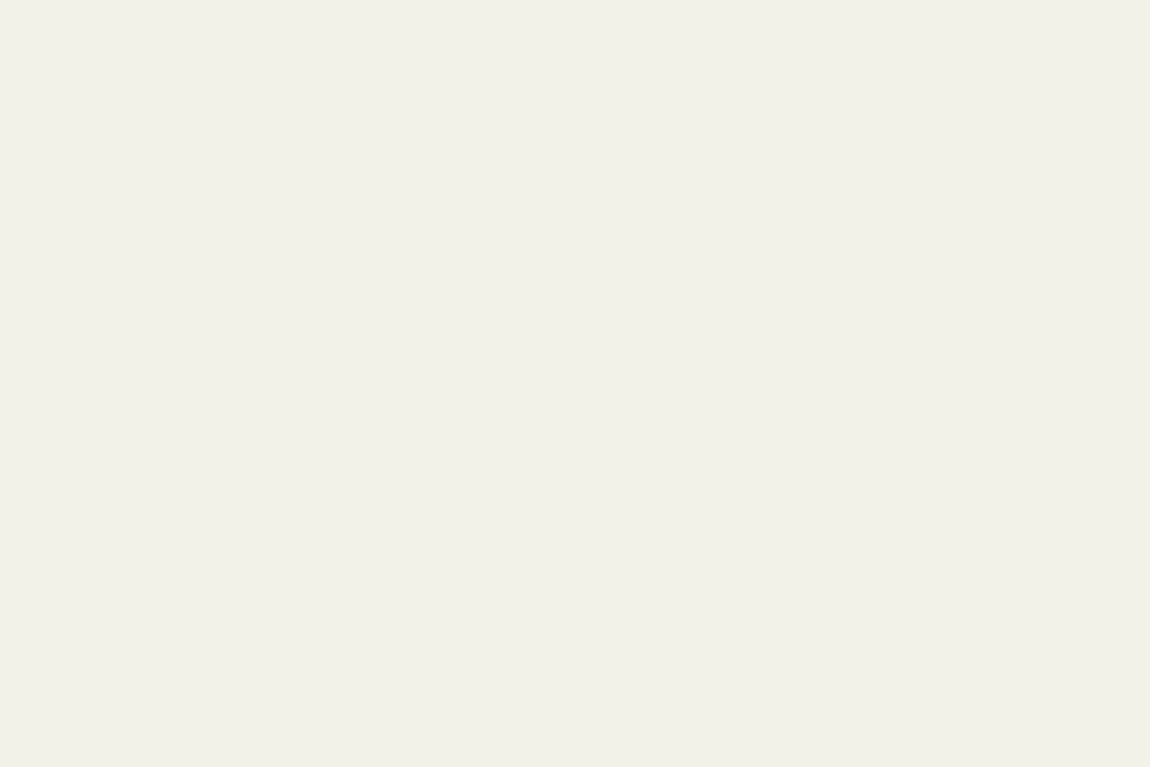
Learn how McDonald’s combines innovation, sustainability, and strategy to thrive globally. Explore insights from this McDonald’s case study for business studies